Advancing Explainable AI for a Responsible Future
Added: January 10, 2025
As artificial intelligence (AI) becomes increasingly integrated into our daily lives, ensuring these systems are transparent and trustworthy is more crucial than ever. Explainable AI (XAI) provides the foundation for building systems that not only perform effectively but also inspire confidence in their users.
To fully realize this potential, the field must address key challenges and opportunities:
1. Developing Stronger Frameworks for Explainability
Many AI systems still lack the robust structures to ensure fairness, transparency, and trust. Current methods, such as feature-based explanations, often fall short of delivering meaningful insights. Addressing this gap requires more adaptable frameworks and dynamic taxonomies to meet the demands of diverse applications.
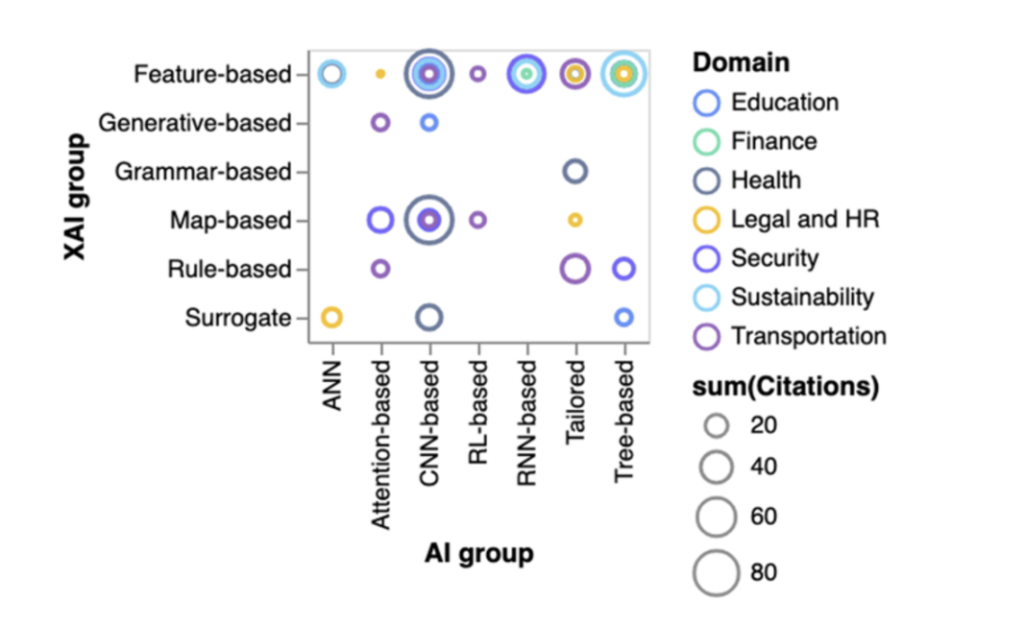
Image 1: Isabel Carvalho, CISUC
2. Enhancing Explainability in Large Language Models (LLMs)
Large Language Models (LLMs) present unique challenges in interpretability due to their complexity and scale. While they excel at generating human-like responses, understanding how they arrive at their decisions is often opaque. Dialogue flow discovery has emerged as a promising approach to tackle this issue, helping validate conversational paths, interpret model outputs, and ensure alignment with intended behaviors. Advancing interpretability tools and techniques specifically designed for LLMs will be crucial to harnessing their potential responsibly.
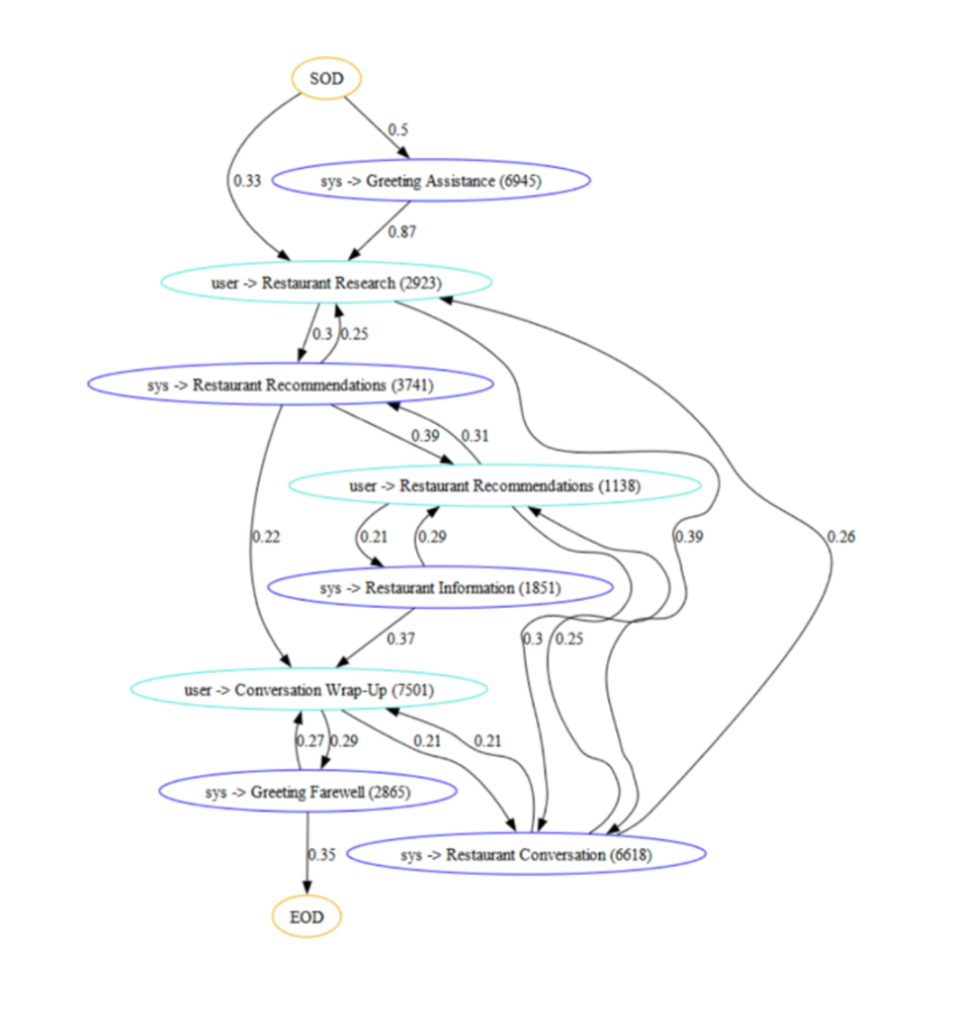
Image 2: Patrícia Ferreira, CISUC
3. Recognizing the Context-Dependent Nature of Explainability
Explainability is not a one-size-fits-all solution. Its significance varies across contexts, becoming critical in sensitive applications such as medical diagnostics or autonomous driving. Tailored approaches that account for these variations are essential to ensure XAI systems deliver value where it matters most.
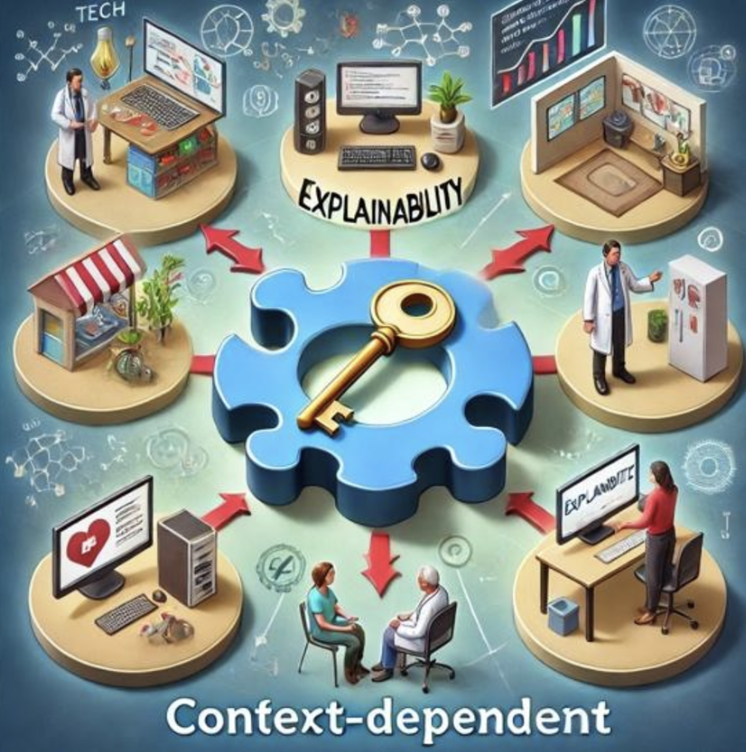
Image 3: DALL-E 3
4. Enabling Adaptive and Context-Aware Explanations
New frameworks like PonderXNet, by Automaise, are pushing the boundaries of concept-based explanations, introducing adaptive computation tailored to specific contexts. These advancements pave the way for AI systems that can dynamically adjust their explanations to suit user needs and improve decision-making processes.
5. Bridging the Gap Between Awareness and Action
Industry awareness of the need for explainability is growing. It is becoming more common for organizations to invest in tools and methodologies aligned with responsible AI practices. However, bridging the gap between awareness and actionable, scalable solutions remains a challenge that demands continued collaboration between researchers, developers, and stakeholders.
Building a Transparent and Trustworthy AI Ecosystem
Advancing explainable AI is a collective effort that requires innovation, cross-disciplinary collaboration, and a user-centric approach. By focusing on adaptable frameworks, context-sensitive solutions, and actionable tools, we can create AI systems that serve society responsibly and transparently. As AI continues to shape our world, investing in explainability will be critical to ensure its benefits are equitably distributed and universally trusted.